Go back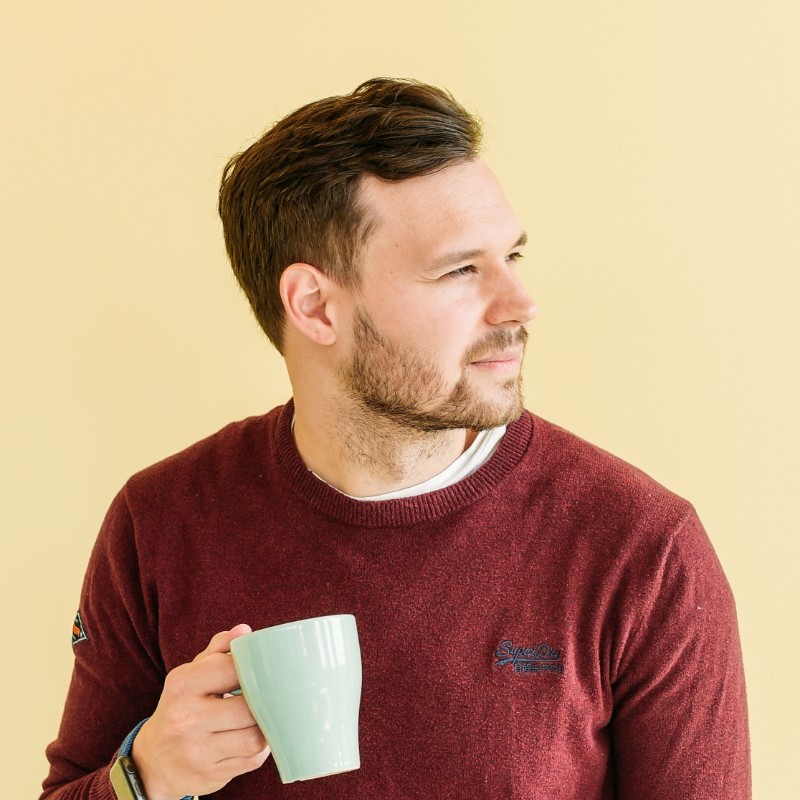
Pioneering Innovation: How GenAI is disrupting Finance & Banking - with free ebook download
An actionable guide to the diverse elements of Generative AI in Finance and Banking that are changing the landscape of productivity, customer experience, and the way the industry operates.
Jason Wirth
3/6/2024
Let’s explore the origins and history of Generative AI, clarifying the current buzzwords you often hear and their meanings. Additionally, we’ll examine real-world applications and use cases that industry leaders are currently exploring, developing, and implementing.
Exploring the Core Concepts of AI
Check out our glossary for a quick overview of what these buzzwords mean, or download the eBook for descriptions and more.
The Evolution of AI: A Quick Recap
1950s: The Dawn of AI - The era began with Alan Turing proposing the Turing Test.
1980s: Neural Networks - Introduced by John Hopfield with the Hopfield Network, showcasing the problem-solving potential of neural networks.
1990s: Machine Learning Evolution - Marked by IBM’s Deep Blue defeating world chess champion Garry Kasparov.
2000s: The Rise of Deep Learning - Geoffrey Hinton and his colleagues introduced the concept of “Deep Learning” to describe neural networks with many layers.
2010s: Breakthroughs in Generative AI - Highlighted by Ian Goodfellow introducing Generative Adversarial Networks (GANs) in 2014 and the introduction of GPT (Generative Pre-trained Transformer) by OpenAI in 2018.
2020s: Advancements and Mainstream Adoption - GPT-3 was released by OpenAI, showcasing significant improvements in language understanding. Then, in 2024, GPT-4 was released, changing the world.
2024: Your Journey Begins - Rapid enterprise GenAI adoption across industries.
Examining the Hype of GenAI: Is It Real?
Don’t just take our word for it; major research institutions have published reports supporting GenAI’s significant potential in the industry.
77% of financial service providers believe AI will be essential to their business within two years, according to Cprime.
$200 to $340 billion in value added to the banking sector thanks to improved productivity, according to MIT Technology Review Insights.
The Tangible Benefits of GenAI Adoption
Knowledge Gap Solved - By shortening the cycle to first usage, you can quickly build a “blueprint” of what works.
Teams Being Supported - With a clear scope, you can launch faster and see the business impact quickly.
Improved Customer Service - Involving domain experts during development simplifies change management.
Diving into Real-World Use Cases & Applications
Customer Service & Support - GenAI enhances customer service with rapid query resolution, personalized information retrieval, and proactive support, promising a seamless and improved customer experience.
Risk Management & Compliance - GenAI automates and improves compliance and risk management by predicting issues and ensuring regulatory adherence, securing operations efficiently.
Operations and Back-Office - GenAI boosts back-office efficiency by automating tasks and data analysis, cutting costs, and aiding strategic decision-making.
Marketing and Sales - Generative AI enhances marketing and sales through automated content creation, personalized interactions, and insightful data analysis, leading to improved efficiency and increased revenue.
Powerful applications finance and banking enterprises are exploring include:
- Automatic Extraction of Financial Data from Financial Reports
- Enhanced Fraud Detection and Prevention
- Automated Financial Advising
- Internal Customer Service Conversational AI
- Automated loan approvals
- Generating financial reports
- News analytics
- Know your customer (KYC)
- Sales assistant
- Contract intelligence
Connect Your Data to LLMs with RAG
When considering the development of Generative AI applications, our priority is to prepare the data in a way that allows the application to deliver the desired outcomes. To facilitate this, we emphasize the importance of a concept called Retrieval Augmented Generation (RAG). Implementing an effective data-enriched RAG process significantly enhances the likelihood of success for your AI application.
This addresses several key factors contributing to the failure of General AI, particularly the erosion of confidence caused by the low accuracy of responses, often a result of the quality of data input. Your AI is only as good as your data.
The free eBook
Sign up for our newsletter to get the latest updates on LLM.
Go to newsletterSee it in action.
Related use cases.
Learn more about this in our use cases.