Go back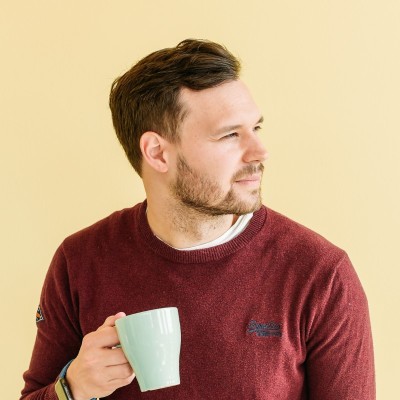
4 Key components of a success RAG pipeline for your GenAI application
Learn about the key elements of a successful RAG pipeline for Generative AI, focusing on effective data organization, advanced AI techniques, and continuous quality monitoring to enhance AI response accuracy and relevance.
Jason Wirth
4/18/2024
What does a successful RAG pipeline result in?
Improved Accuracy and Relevance of Responses
The primary results of a successful RAG pipeline is the considerable improvement in the accuracy and relevance of the generated response from your model. By incorporating data-centric RAG techniques the chances that the final responses are not only precise but also contextually relevant
This is particularly beneficial in domains requiring high levels of specificity and customization powered by complex data models.
Enhanced User Experience
User satisfaction is directly influenced by the interaction quality they experience. A RAG pipeline ensures that responses are not only relevant and accurate but also detailed and informative, significantly enhancing user engagement. This can lead to increased user retention and higher satisfaction rates, required for enterprise GenAI adoption.
Scalability and Flexibility
A successful RAG pipeline allows for scalability and flexibility in applications. As the demand for AI services grows, the system can expand without a degradation in performance. This scalability is crucial for applications with a growing user base or those requiring real-time responses across various topics. Additionally, the flexibility offered by a robust RAG pipeline means that the system can adapt to different domains or integrate new data sources with minimal reconfiguration.
Cost-Effectiveness
While the initial setup of a RAG pipeline might requires a certain investment in terms of time and resources, the long-term benefits include reduced operational costs. By automating critical elements of your operational processes and enhancing the precision of outputs, there is less need for human intervention in managing responses, thereby reducing labor costs and minimizing errors.
4 Important component of a successful RAG pipeline
1. Focus on the underlying data: Model a mindmap-like data structure
The first step in improving a RAG pipeline is to organize data sources into a mindmap-like structure. This method clarifies complex relationships and enhances the retrieval process, leading to more accurate and relevant AI responses.
A mindmap is a visual representation that mimics the way the human brain organizes and processes information. It is inherently designed to highlight connections between different pieces of information, making it an ideal tool for managing the vast and varied datasets used in RAG systems.
2. Inferring Filters from the Question
To enhance the precision and relevance of responses in a RAG pipeline, our approach utilizes advanced AI technologies, specifically customizable intent AI and psychology AI. These technologies play a crucial role in accurately determining the user's intent.
Intent AI - It looks beyond the superficial layer of words to understand the purpose and expectations embedded in the user's question. For instance, when a user asks, "How can I improve my sleep quality?", the intent AI discerns not just a query about sleep, but a request for solutions that enhance sleep quality.
Psychology AI - Complementing the intent AI, psychology AI delves into the emotional and psychological states suggested by the user’s language. This aspect of AI assesses tone, urgency, and underlying emotions to tailor responses
Once the intents and psychological contexts are inferred, the system uses the mindmap-like structure to filter data effectively. The mindmap serves as a dynamic blueprint where each node represents a cluster of related data.
3. Quoting the Data
Once the data has been effectively filtered using our mindmap-like structure and AI-inferred filters, the next crucial step in our RAG pipeline is to present this data to the user in a clear and validated manner. To achieve this, we employ sophisticated language models (LLMs) like ChatGPT designed to quote the data accurately and understandably.
Language models in our system are not merely tools for generating text; they are also configured to understand and encapsulate the essence of the retrieved data. This involves translating complex data points into user-friendly language that maintains the integrity and accuracy of the information.
The process of quoting data involves more than reproducing facts; it requires the data to be framed in a context that is relevant to the user’s initial query. This contextualization helps users understand why the information is pertinent and how it relates to their questions.
4. Ongoing, Automated Data Quality Checks
To guarantee the reliability and currency of the data used in our RAG pipeline, we employ a dedicated data quality AI. This technology is critical in continuously verifying the integrity and accuracy of the information our system retrieves and presents.
---
Data-centric RAG is an iterative approach from chatbot to agent/automation, enabling LLMs to deeply comprehend a company’s fundamental products and services, along with their interconnections. As a result, it enhances the LLM’s ability to execute tasks with remarkable reliability.
Keep on learning about RAG:
Episode 1: Introducing Data-Centric RAG: Powering the Most Reliable and Accurate GenAI Applications - read here
Sign up for our newsletter to get the latest updates on LLM.
Go to newsletter